The Importance of Storytelling in Data Visualization
- Avdhesh Gaur
- Nov 6, 2019
- 5 min read
Updated: Nov 11, 2024
Presenting the findings based on our analysis is as important as coming up with these fruitful insights. Even though the same can be taken care of in multiple ways, but Storytelling certainly provides you and the audience the opportunity of active participation.
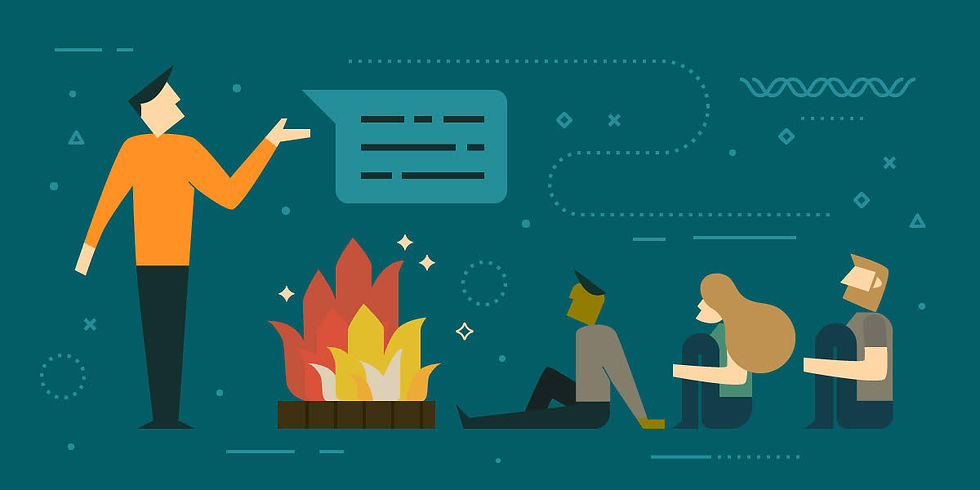
A great story is a “Show Me” story & not a “Tell Me” story. Instead of saying, 20% of people are poverty-stricken. (That might have an impact on you. But odds are you won’t really remember it three days from now)
What if we say, I found out that poverty has impacted our society so much that, 1 out of every 5 people is affected by the same, marking a significant 20% poverty rate. Out of every 100 people, 20 are not even able to afford the basic necessities. (The possibility of triggering the audience’s cognitive empathy is quite higher in this case & so are the chances of remembrance) Stories activate the same parts of our brains that are activated when we are processing a real experience.
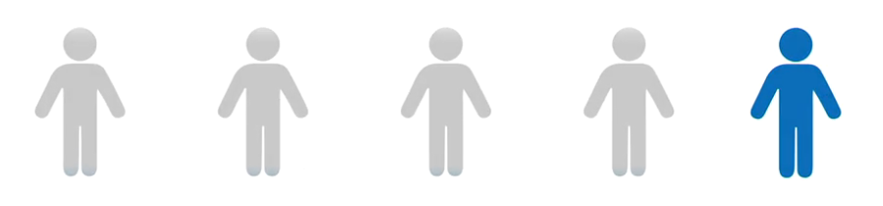
Going back in time, thinking about the evolutionary imperative, many early stories were documentaries, not fiction. Like when ancient human went out on a hunt and came back to the cave to report the results, he/she told stories, it was a compelling narrative, and yes, it contained data, right?
The story would explain what time the hunting party left, what direction they traveled, where they found their prey, how many miles they ran, etc. But they were telling it in a story form, they didn’t have a spreadsheet, it was always a story. Humans evolved to produce and consume stories as a survival mechanism. This is why storytelling does create a substantial difference, while we present some interesting discovery.
The 4x4 approach
Some stories are simple, while the other times you need to present higher-level information to people to get them to self-select in for deeper and more complex content, based on their interest in the subject matter.
This brings up a 4 x 4 Approach. It’s well known as Water Cooler, The Cafe, The Research Library, and The Lab conversation.
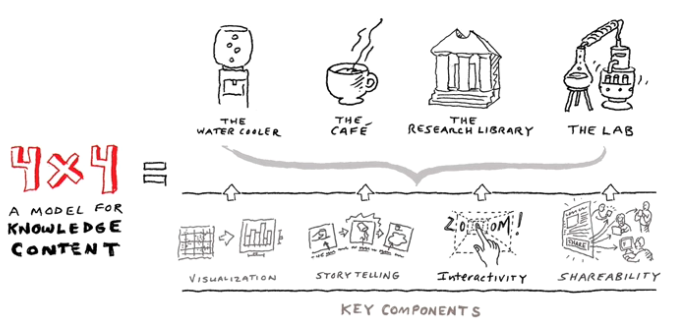
The Water Cooler moment is essentially that initial attention-grabbing piece of content. It’s the thing that lets people decide whether or not they’re interested in what you’re talking about. Just like if you’re at the water cooler, they’ll decide if they want to hang out and talk longer. If they do want to talk longer, they’ll go to the cafe with you and have a cup of coffee. So The Water Cooler moment is like the image or the headline. The Cafe content is like the blog post or the short article, three to five-minute video. If they’re really still interested in the topic, they’ll go to the research library.
They will dig deep. They will read the 100-page PDF. And finally, you have The Lab experience, which is like that interactive data experience, where people can explore the content much more deeply and maybe on their own. Those are the four levels of content. The point is that, whether you create Research Library or Lab-level content, however deep you do go, you always have to create Water Cooler content first, followed by Cafe content, followed by whatever comes after that. It’s all about stepping people into content and letting them self-select based on their interest in what you are talking about.
The Eye candy
Eye candy can complement a story along with the visualization. There’s an ongoing argument that plays out in this corner of the universe about the validity of using eye candy to keep things interesting, so we entertain as well as inform. I fall clearly on the side that acknowledges that as communicators we must entertain as well as inform. But of course, it goes without saying that this entertainment can’t come at the expense of accuracy and truthfulness in our data communications. For instance, you shouldn’t pick fancy charts because they’re more interesting than a bar chart unless they’re also at least equally as accurate, understandable, and clear.
Repetition
Repetition and redundancy in data stories are a compelling tool. Recent research has found that we can achieve measurable increases in the memorability of data visualizations with certain types of repetition and redundancy. This redundancy comes in three primary types. First, there is message redundancy, which is when the main conclusion or message is presented in multiple ways. But it’s not just about labeling. That same research report found that charts that include a title that explains what the chart’s about are much more memorable than those without titles, or with titles that aren’t explaining the message of the visualization.
Then there’s data redundancy, which is when the data itself is presented in multiple ways. Finally, there’s the repeated use of visual metaphors, and by this I mean the reuse of visual cues let your audience understand what they’re looking at and what it all means.
Another technique is the thoughtful use if repeated visual metaphors. When you repeat the use of certain shapes, colors, and chart forms throughout a piece, you allow your audience to learn something new once, and then reinforce that learning throughout, which will increase the understanding of your story and has the added benefit of improving their memory as well. You’ve probably heard the aphorism about how to present something. First, tell them what you’re going to tell them, then tell them, then tell them what you just told them, or the idea that you have to see an ad seven times before it will have an effect on you.
A Story is 22 times more memorable than facts alone: Jennifer Aaker
In General, We need a story, we expect a story. We recognize what makes up a story, so when you’re communicating, you have to think like a storyteller, especially when you’re communicating data, which is inherently not anxiety-producing, and does not produce any emotion or evolutionary imperative. The data means almost nothing without a context, without the story form around it. So communicating data in a story is necessary, natural, and essential.

Avdhesh Gaur has 5+ years of work experience with Tableau desktop, taking down various business requirements by building Data-driven Business Intelligence models using SQL, Hive, Tableau, and other Statistical data analysis Techniques.
In a nutshell, He transforms data into visualizations to help organizations understand their business operations more visually.
Avdhesh got one of his submissions to Tableau accepted & placed in their permanent public knowledge database known as "Tableau Gallery" on 7th January 2019, which in a more cooler way we call Viz of the day
He is a featured author at O'Reilly's - Safari Books Online. "Data Visualization with Tableau in Practice" is the recent video course that he had worked on in collaboration with Packt Publishing. The same was released by the End of April 2019.
What you will find in this course?
댓글